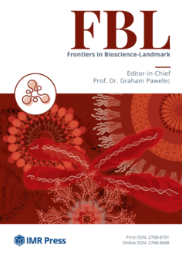
Frontiers in Bioscience-Landmark
(Print ISSN: 2768-6701 | Electrronic ISSN: 2768-6698)
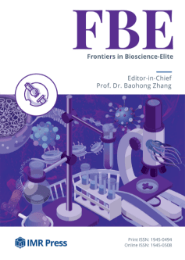
Frontiers in Bioscience-Elite
(Print ISSN: 1945-0494 | Electronic ISSN: 1945-0508)
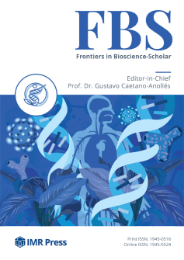
Frontiers in Bioscience-Scholar
(Print ISSN: 1945-0516 | Electronic ISSN: 1945-0524)
The journals' website domain has been updated. Kindly click on the link below:
https://www.imrpress.com/
https://www.imrpress.com/